BLOG
Building a Scalable Digital Core with Platform Engineering for AI
Discover how platform engineering empowers enterprises to scale AI securely and efficiently. Learn the key capabilities, challenges, and strategies to build a future-ready digital core.
April 21, 2025

Introduction
What’s stopping most AI strategies from scaling beyond prototypes? It’s not ambition. It’s architecture.
From customer service chatbots to generative product design, enterprises are racing to integrate AI across the value chain. Yet, despite aggressive investment, the gap between experimentation and enterprise-wide impact remains wide.
According to Deloitte’s 2024 State of Generative AI in the Enterprise report, 68% of organizations say that 30% or fewer of their GenAI experiments have been fully deployed into production. The appetite for AI is high, but the infrastructure to support it often falls short.
This isn’t a tooling problem. It’s a systemic one. And solving it requires a fundamentally different approach to enterprise infrastructure: one that is composable, intelligent, and built for rapid iteration and scale.
That’s the promise of platform engineering. More than a DevOps evolution, it’s the strategic foundation of AI-first organizations. At its center lies the digital core—a unified platform that brings together data, compute, APIs, and observability into a seamless, scalable engine for intelligent operations.
Let’s explore what this digital core looks like—and how to build it for sustainable AI success.
Defining the Digital Core: Beyond Infrastructure
Th/e digital core is the foundational layer of modern enterprise systems. It's what enables agility, intelligence, and resilience at scale—not just in infrastructure, but in how organizations operate and evolve. Think of it as the AI-ready backbone of the business.
Core Elements of a Digital Core
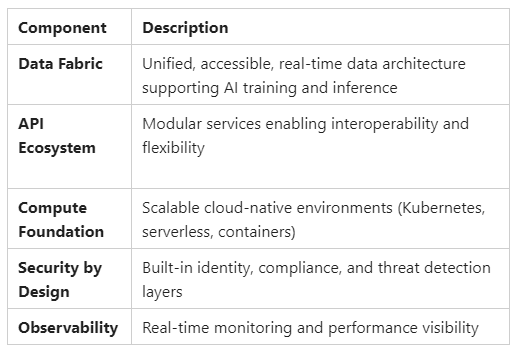
Traditional infrastructure models—built around monolithic systems or disconnected tools—lack the flexibility to support modern AI workloads. Instead, a composable, service-oriented architecture allows AI models to plug into and interact with dynamic data streams, compute services, and business logic.
A scalable digital core also reduces time-to-market. By enabling modular development and deployment, it lets organizations move from experimentation to real-world value faster—critical in the high-velocity AI era.
Platform Engineering: The Enabler of Enterprise AI
Platform engineering is the discipline of building and maintaining internal developer platforms (IDPs) that serve as a product for AI, data science, and DevOps teams. These platforms abstract infrastructure complexity and deliver self-service environments for experimentation, deployment, monitoring, and scaling.
Key Benefits of Platform Engineering for AI
- Velocity: Enables faster iteration and deployment cycles for AI models
- Governance: Centralizes controls, security, and compliance
- Standardization: Promotes reusable components and shared practices
- Collaboration: Breaks down silos between engineering, data science, and operations
- Resilience: Ensures platform reliability under growing AI workloads
According to Gartner, by 2026, 80% of software engineering organizations will establish platform teams as internal product providers for developers, data scientists, and engineers.
Platform engineering isn’t a siloed practice—it’s a strategic shift in how enterprises build digital capabilities. When aligned with AI objectives, it becomes the foundation for scalable intelligence.
Core Capabilities for an AI-Ready Platform
To scale AI from isolated experiments to business-critical applications, enterprises need more than piecemeal solutions—they need a purpose-built, composable platform. This digital core must integrate the following foundational capabilities to ensure speed, security, and scalability:
1. Infrastructure as Code (IaC): Codifying Consistency and Agility
Manual infrastructure provisioning is error-prone and inconsistent. IaC transforms infrastructure into version-controlled, repeatable code—enabling rapid environment setup across hybrid or multi-cloud systems.
- Automates the provisioning of compute, networking, and storage across public cloud and on-prem.
- Ensures consistent environments across dev, test, and prod pipelines.
- Tools: Terraform, Pulumi, AWS Cloud Development Kit (CDK)—enabling declarative provisioning that aligns with compliance and DevSecOps best practices.
2. MLOps Pipelines: Operationalizing the AI Lifecycle
MLOps brings DevOps principles to the machine learning lifecycle, enabling continuous integration, delivery, and governance of AI models.
- Automates end-to-end workflows: from data prep and training to deployment and monitoring.
- Integrates tightly with CI/CD, version control, and observability tools.
- Supports intelligent rollouts—like shadow testing, canary releases, and automated rollback—reducing risks in production environments.
3. DataOps & Unified Data Access: Making Data AI-Ready
AI is only as good as the data it learns from. DataOps ensures data availability, quality, and governance—turning messy data into trusted fuel for intelligence.
- Enables secure, governed access to real-time, labeled, and structured data.
- Centralizes metadata through data catalogs and automates lineage tracking for transparency and compliance.
- Integrates with modern data stacks: data lakes, warehouses, and streaming platforms like Kafka and Delta Lake.
- Enhances collaboration across data scientists, engineers, and business users.
4. Cloud-Native Architecture: Scaling AI with Modern Infrastructure
Traditional monolithic infrastructures can’t support AI’s dynamic workloads. Cloud-native design unlocks elasticity, resilience, and performance.
- Leverages Kubernetes, containers, and microservices to enable horizontal scalability and modular deployments.
- Supports serverless architectures for event-driven processing and real-time AI inference.
- Optimized for GPU, TPU, and AI accelerators to handle compute-intensive model training and deployment.
5. API-Driven Ecosystems: Modular Intelligence Across the Stack
An AI platform thrives on interoperability. APIs enable modular AI services to interact seamlessly across systems and teams.
- Facilitates integration of internal models and external AI services (e.g., LLMs, analytics engines).
- Encourages reuse of AI capabilities—like recommendation engines or fraud detection—via shared API endpoints.
- Powers cross-domain intelligence by enabling different applications to exchange real-time insights.
6. Built-in Observability & Compliance: Trust, Transparency, and Governance
As AI models scale, so do risks. Observability ensures performance reliability, while compliance frameworks mitigate security and regulatory exposures.
- Embedded monitoring using tools like Prometheus, Grafana, Datadog, and OpenTelemetry.
- Real-time alerts for model drift, latency spikes, and data anomalies.
- Granular role-based access control (RBAC), full audit trails, and support for automated compliance with frameworks like GDPR, HIPAA, and SOC 2.
Challenges in Building the Digital Core—And How to Overcome Them
Developing a digital core that can support AI at enterprise scale is a transformative effort—and like any transformation, it comes with real complexity. But these challenges are navigable with the right strategy, capabilities, and leadership alignment.
1. Integration with Legacy Systems
The Challenge:
Many enterprises are still running critical operations on legacy systems that were never built to handle real-time AI workloads, microservices, or elastic scaling. These systems often create data silos and limit interoperability.
The Solution:
Adopt a gradual transformation approach. Use abstraction layers like APIs and event-driven architectures to modernize without full system replacement. Decompose monolithic systems incrementally, enabling coexistence with cloud-native services during the transition.
2. High Initial Investment
The Challenge:
Establishing a robust internal platform often requires upfront investment in automation, observability, and integration tooling. Without immediate ROI, such foundational work may face resistance from budget owners.
The Solution:
Prioritize scalable wins. Focus on high-value, low-risk AI use cases—like forecasting, anomaly detection, or process automation—that can be piloted quickly. Build the platform around these to prove value. Optimize costs by leveraging elastic infrastructure, open-source tooling, and modular design principles.
3. Security, Compliance & Governance
The Challenge:
The more distributed, data-intensive, and dynamic the platform becomes, the more complex its security and compliance requirements. AI introduces unique risks like model drift, adversarial attacks, and data lineage opacity.
The Solution:
Bake in security from the start. Establish identity-based access controls, real-time monitoring, and automated audit trails. Enable continuous compliance checks tied to regulatory and ethical standards. Integrate explainability, fairness, and bias detection into AI model governance to meet internal and external accountability requirements.
Conclusion: Building for AI Scale Starts at the Core
AI is transforming how enterprises create value—but only if the foundation is ready to scale. Platform engineering is the enabler. It allows enterprises to build reusable, secure, and intelligent environments that reduce friction and accelerate transformation.
The digital core makes the difference between isolated AI use cases and enterprise-wide intelligence. The organizations that win in the next era will be those that invest now in building scalable, composable, and resilient platforms that power continuous innovation.
At Clarient, we help future-focused enterprises design and build AI-native digital cores—from infrastructure blueprints to platform enablement. Whether you’re scaling GenAI models, building internal developer platforms, or transforming your MLOps architecture, we bring the expertise, frameworks, and speed you need to lead. Let's Talks!

Parthsarathy Sharma
B2B Content Writer & Strategist with 3+ years of experience, helping mid-to-large enterprises craft compelling narratives that drive engagement and growth.
A voracious reader who thrives on industry trends and storytelling that makes an impact.
Share
Are you seeking an exciting role that will challenge and inspire you?
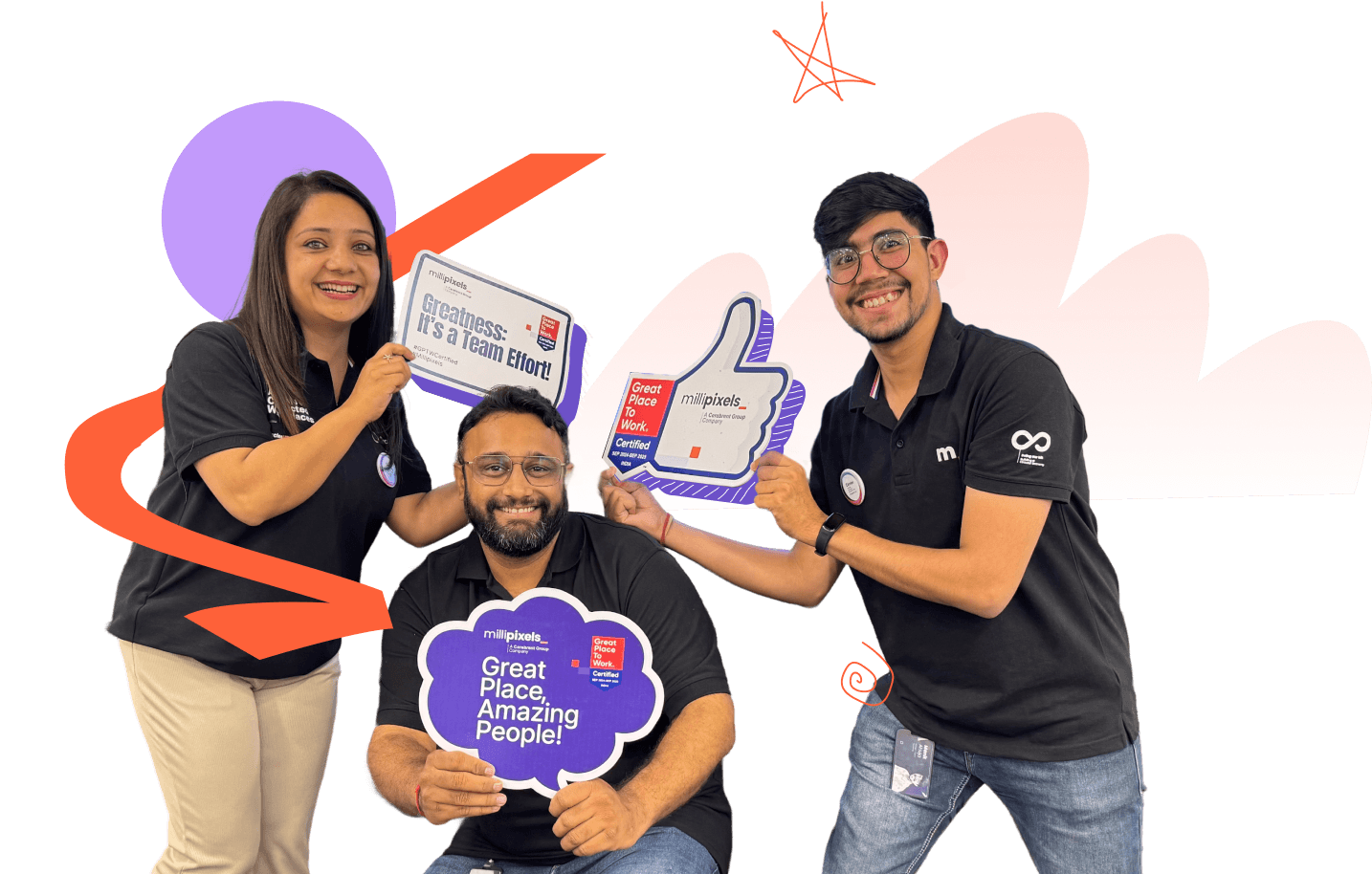
GET IN TOUCH